Rethinking Mission-Capable AI: How to Overcome the Biggest Barriers for Government Adoption
April 30, 2025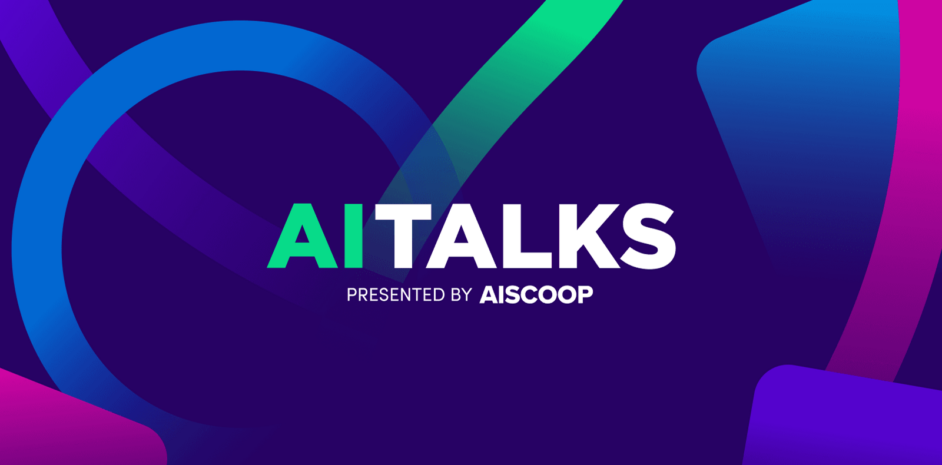
At Seekr’s first AITalks last week, Seekr President Rob Clark delivered a keynote on “Advancing AI Transformation Agencywide”, where he highlighted the four barriers to AI adoption, and how to address them to build mission-capable AI.
Clark started by sharing Seekr’s origin story. Seekr was founded four years ago after Seekr’s founders discovered an alarming trend in the amount of biased information and content that people were consuming. With the explosion in use of AI technologies, foundation models are being built with public information from the internet, loaded with bias, misinformation, and disinformation. These models are being trained on popular information, not credible or vetted information. So Seekr forged a different path—one that emphasized data provenance, lineage, with greater transparency, explainability and contestability for Generative AI.
Today, Seekr’s enterprise AI platform empowers the Department of Defense, so mission owners can safely build and deploy trusted Large Language Models (LLMs) and AI Agents using their own vetted agency data, wherever it lives, and on any infrastructure of their choice. Seekr AI supports multiple mission-critical use cases, including explainable LLMs, bias mitigation, situational awareness, and even weapons system vulnerability testing. And in areas like supply chain risk and fraud prevention, Seekr has helped agencies evaluate threats, perform due diligence on supply chains, and empower analysts to produce reports in minutes instead of weeks—augmenting, not replacing, human judgment.
By working with highly regulated customers like the DoD, Seekr isolated four key barriers to agencywide AI adoption: Trust, Data, Talent, and Portability.
1. The Trust Problem
Trust is the foundation for everything, not a badge or a label one bestows on oneself. It is also about accuracy—models that avoid producing hallucinations or plausible but incorrect responses (we’ve all seen how confidently wrong ChatGPT can be!). That means no hallucinations, no data leaks, and compliance with a variety of security frameworks.
Trust is also about transparent, measurable, and explainable AI that comes with guardrails, ensuring safety and privacy, conforming to security frameworks, and protecting data sovereignty. The House Intelligence Committee recently reported on the frightening number of commercial AI systems that are sharing highly sensitive, proprietary information with America’s adversaries — contracts, documents, personal records, financial data. Rightfully, they have demanded immediate action to safeguard the security and integrity of government systems.
Today, agencies can accelerate and run faster when they can understand what an AI system is doing and why it is doing it. Data Point Attribution is critical and allows agencies to ask models to show them where and what in the training data is causing certain responses or actions, prove models comply with relevant compliance frameworks, and measure how well models align with mission priorities. Afterall, how can you properly audit AI systems if they cannot explain themselves?
Agencies should look for partners and software that will always tell them the ‘why,’ with native tools for transparency, explainability, and measurability from beginning to end.
2. The Data Problem
No foundation model addresses government needs out of the box. Therefore, models must be trained and configured with mission-specific data, so they are relevant and generate responses based on domain expertise. That starts with preparing clean, labeled, AI-ready datasets that impart domain expertise to models.
Today, agencies can accelerate data preparation using automated Agentic AI—no PhDs or data engineers required, to create reliable, transparent data pipelines to feed the AI systems.
Agencies should seek out commercial products that accelerate and simplify the path to clean, credible, reliable data, and solve the labeling problem.
3. The Talent Problem
Finally, the AI Workforce skill gap is real. Agencies struggle to compete with the private sector for top AI talent, and traditional hiring processes have not always kept pace with the skills needed to deploy and manage modern AI systems. At the same time, it must better leverage the employees they do have, boost productivity, and help more of them build and deploy AI that assists them with jobs that humans cannot do, or basic tasks they do not want to do.
A 2024 Ernst & Young study notes that half of the federal workforce is using some kind of AI, proving that the demand is there. Unfortunately, most employees use AI to boost productivity, not for solving true business and mission problems. Human-machine teaming has the potential to be a force multiplier if the right tools and safeguards are in place.
Agencies must empower more of their workforce—analysts, mission owners, IT—to build with AI (beyond chatbots), while data scientists handle last mile data analysis.
4. The Portability Problem
AI software must meet agencies where they are at and remain agile, so agencies can deploy AI across contested, degraded, and disconnected environments. Ideally, agencies should centrally manage AI and bring it to where data resides, whether that’s on-premises, in multiple clouds, or on edge devices.
Finally, an agency’s data, IP and algorithms should remain accessible, not locked in a black box or a multi-billion-dollar contract, that forces them to pay for the privilege of accessing these valuable assets.
Conclusion
We know AI can transform missions—but only with tools built for trust, accuracy, and agility. With faster procurement and transparent systems, we can move faster than our adversaries and reimagine a government that is AI-powered, nimble, and trustworthy.
Explore trusted AI solutions for government
Learn More