Why Responsible AI Is the Smart Path to Scalable Innovation
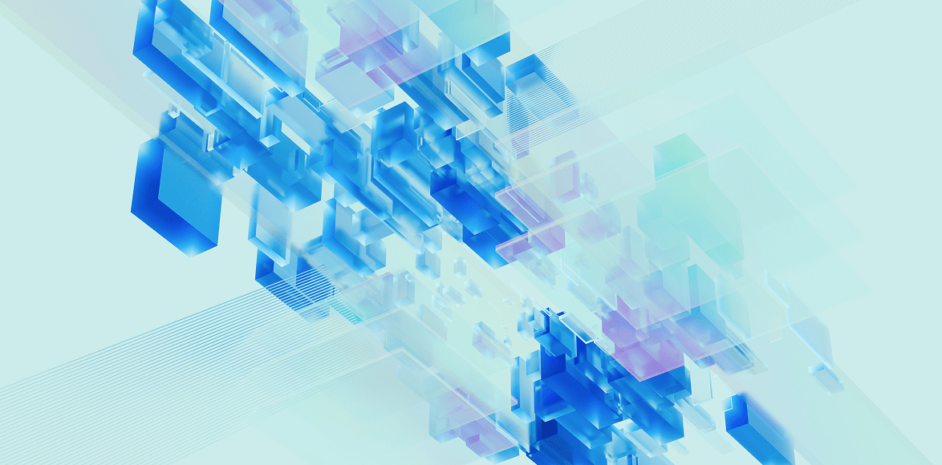
Key takeaways
- Responsible AI promotes fairness and equity in real-world applications. By scrutinizing your training data and model outputs for bias and unfairness, you can enable better outcomes for everyone.
- Responsible AI starts with responsible data. Flawed data leads to flawed outputs, which can damage trust and cause real-world harm.
- The definition of responsible AI depends on your organization. Each enterprise needs to define what ‘responsible’ means in the context of their overall mission and principles.
- Strong data governance and transparency lay the foundation for building responsible and trustworthy AI systems. Building a thorough data management and control framework for your organization can help prevent the wrong data from being used for training.
- Simplify your path to responsible AI with an end-to-end platform that provides transparency into model reasoning, robust explainability tools, and intelligent, high-quality dataset generation in one place.
What is responsible AI?
While deploying AI is a strategic imperative, real enterprise value comes from systems that produce fair, trustworthy outputs aligned with company principles and ethical standards. Without clear safeguards to ensure ethical outputs, organizations can accidentally cause harm, violate laws, or fail to live up to their own values. Responsible AI isn’t just a checkbox exercise—it’s the foundation for lasting trust and a competitive advantage in enterprise innovation.
The core concepts of responsible AI include:
Fairness: Are model outputs equitable and free from bias?
Explainability and transparency: Can you explore how model decisions are made and what data influenced them?
Security and privacy: Are you using compliant, nonsensitive, and secure data in model training? Is the AI system protected against malicious action?
Alignment with human and organizational values: Do model behaviors reflect your company’s mission as well as broader ethical standards?
Responsible vs trustworthy AI: What’s the difference?
Trustworthy AI and responsible AI are both essential to enterprise success, yet each addresses different dimensions of model integrity and ethics:
- Responsible AI is AI that’s ethical, fair, and aligned with human values. With a focus on safety and accountability, it seeks to ensure that an organization’s AI solutions align with its cultural norms and principles as well as broader social values, laws, and regulations.
- Trustworthy AI is more focused on how an enterprise builds and maintains an AI system. The end goal is to ensure that a model provides responses that are reliable and accurate in terms of the company’s specific business context. Without reliable outputs anchored in industry expertise, AI can erode customer trust and tarnish your brand reputation.
Responsible and trustworthy AI are interdependent. Together, they reinforce ethical, reliable, and enterprise-ready systems.
Why responsible AI can’t be an afterthought
AI is now involved in high-stakes decisions that affect peoples lives—from healthcare and hiring to finance and public policy. When systems lack responsible design, the consequences can be hard to recover from.
For example, in 2019, researchers found that racial bias present in commercial algorithms used by US healthcare systems led to a reduction of care for Black patients by more than 50 percent.
More recently, a 2024 UNESCO study showed that generative AI systems continue to associate women with domestic terms such as “home” and “family” four times more frequently than men, reinforcing societal gender stereotypes. Male names were connected to words such as “business,” “executive,” “salary,” and “career.”
Responsible AI practices are essential for reducing these risks and ensuring AI systems benefit all users fairly.
How training data makes or breaks AI responsibility
AI models are only as good as the data they’re trained on. Your training datasets are the lifeblood of the AI model, and they inform everything the model does in production. When models are trained on datasets with biased or poor-quality data, they’re highly susceptible to delivering irresponsible outcomes. Whether you’re building a customer-facing application or integrating AI into internal workflows, your training dataset shapes everything—from tone to accuracy to ethical compliance.
How to build more responsible AI systems
Responsible AI is a nuanced topic and strategies will vary across organizations, but these five core steps can help you take control of your development process:
1. Define what responsibility means for your enterprise
Assemble key stakeholders from various departments to understand the potential impacts of your AI efforts—both positive and negative. Create a unique definition of ‘responsible’ for your organization and examine what your AI solutions need to do to live up to your company’s principles and brand reputation.
2. Establish a strong data governance framework
Data governance frameworks can help you better manage the data you use to train and fine-tune models. These frameworks help you sort data into clearly defined categories, ensuring appropriate and high-quality data sources.
Read our guide to data governance for enterprise AI to learn more about creating a framework for your organization.
3. Audit training data
As an extension of creating clear data definitions and criteria for training use, you’ll also want to thoroughly examine the data sources you are using, weeding out anything that doesn’t meet the standards for quality and equity you established in step two. You can also use AI tools to assign metrics such as toxicity or political bias scores to your model outputs to help understand if you’re meeting your responsible AI goals. Additionally, counterfactual testing—where you assess the variance in outputs by changing inputs such as demographics or geographic region—can help you understand the impact of your training data and identify any output biases.
4. Assess security and privacy protections
Keeping data sources protected and secure is another essential part of responsible AI. Be sure to regularly examine your cybersecurity posture and keep a watchful eye on the infrastructure used for storage, training, and deployment. You can use exercises such as adversarial testing (also known as red teaming), which involve third-party or internal security specialists simulating a malicious cyber attack on your organization, to help identify gaps in your AI security strategy.
5. Invest in tools that promote responsible AI development
To build and deploy responsible AI at scale, enterprises need development tools that embed ethical safeguards into every stage of the AI lifecycle. Choose platforms that offer built-in high-quality data generation, explainability, and traceability features to help you stay compliant without slowing down your path to production.
Simplify your path to responsible AI
SeekrFlow is built to help enterprises deploy custom AI applications faster without compromising trust or responsibility. In one end-to-end platform, your team can:
- Transform limited or inconsistent data into structured, AI-ready datasets—without introducing biases or errors
- Ingest a broad range of documents and formats to fine-tune LLMs with your company principles and guidelines
- Understand and validate model decisions through rich explainability tools
- Apply human-in-the-loop feedback and chain-of-thought traceability to reinforce responsible model behavior
You don’t have to choose between AI innovation and responsibility
As your enterprise pursues AI adoption and moves toward the agentic future, keeping a watchful eye on responsibility and trustworthiness is essential to enabling the outcomes you want to achieve. The most successful enterprise AI strategies are built on a foundation of trust, transparency, and ethical decision-making. When you embed responsible practices into your development process from the start, you’re building smarter, more resilient systems that allow you to scale with confidence.
At the center of your responsible AI efforts are the training datasets your models rely on to produce responses. Taking steps to audit your data and create a strong framework for responsibility and governance can help your organization avoid biased and unfair outcomes. Using tools to automate the creation of high-quality training data can help you build responsible models from the start.
As you seek to innovate with AI, let responsibility guide your strategy.